Project
Music BCI - BrainCoder
This is a BrainCoder real-time feedback system. The circle icon on the keyboard is predicting an imagined musical pitch from the EEG feature at that time. The subject is imagining a musical pitch colored in sky blue on the keyboard, with a task to locate the icon at the keyboard. Also, the auditory feedback is present every time the predictions are made(sound is not out here as the subject is listening to it with earphones). This audio-visual feedback is expected to enhance the pitch sensation of the subject which can be used as a training method for the amusics. The feature extraction method and the related journal article are peer-reviewed and in the revising progress. The system development method and feedback generating method will be written in the article too.
Speech BCI
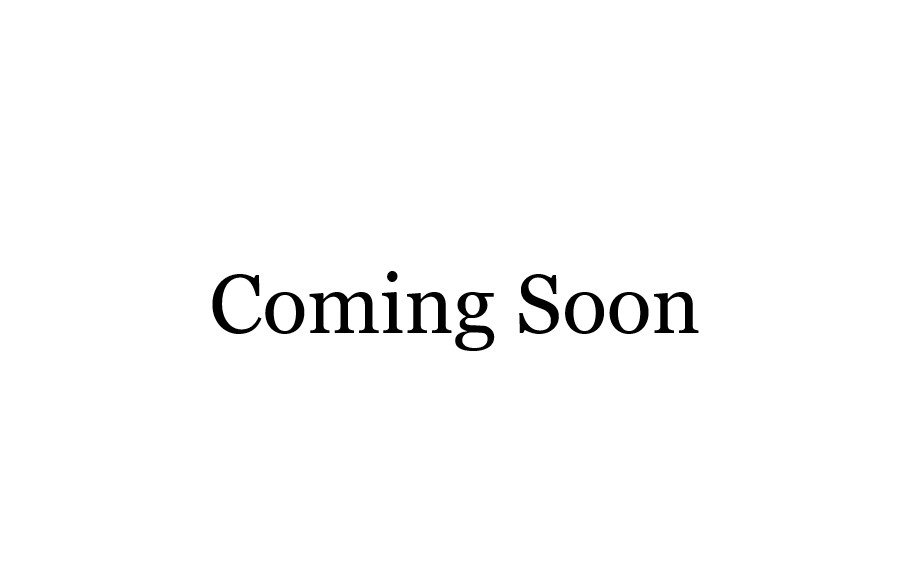
Along with Music BCI, the speech BCI for Korean is in progress. The offline data analysis of Electrocorticogram for 8 Korean words is in the preparation of writing. The content of it is the relationship between the Korean words feature, semantic/phonologic, and the tasks, Covert/Overt, with ERP pattern analysis using DTW(dynamic time warping). However, the final purpose is to generate imagined Korean sentences for patients who lost linguistic communication ability. Currently, I am working on the experiment of Korean words and analyzing them in chunk units, Object and Verb. The image representing the project is upcoming.
AICP Challenge
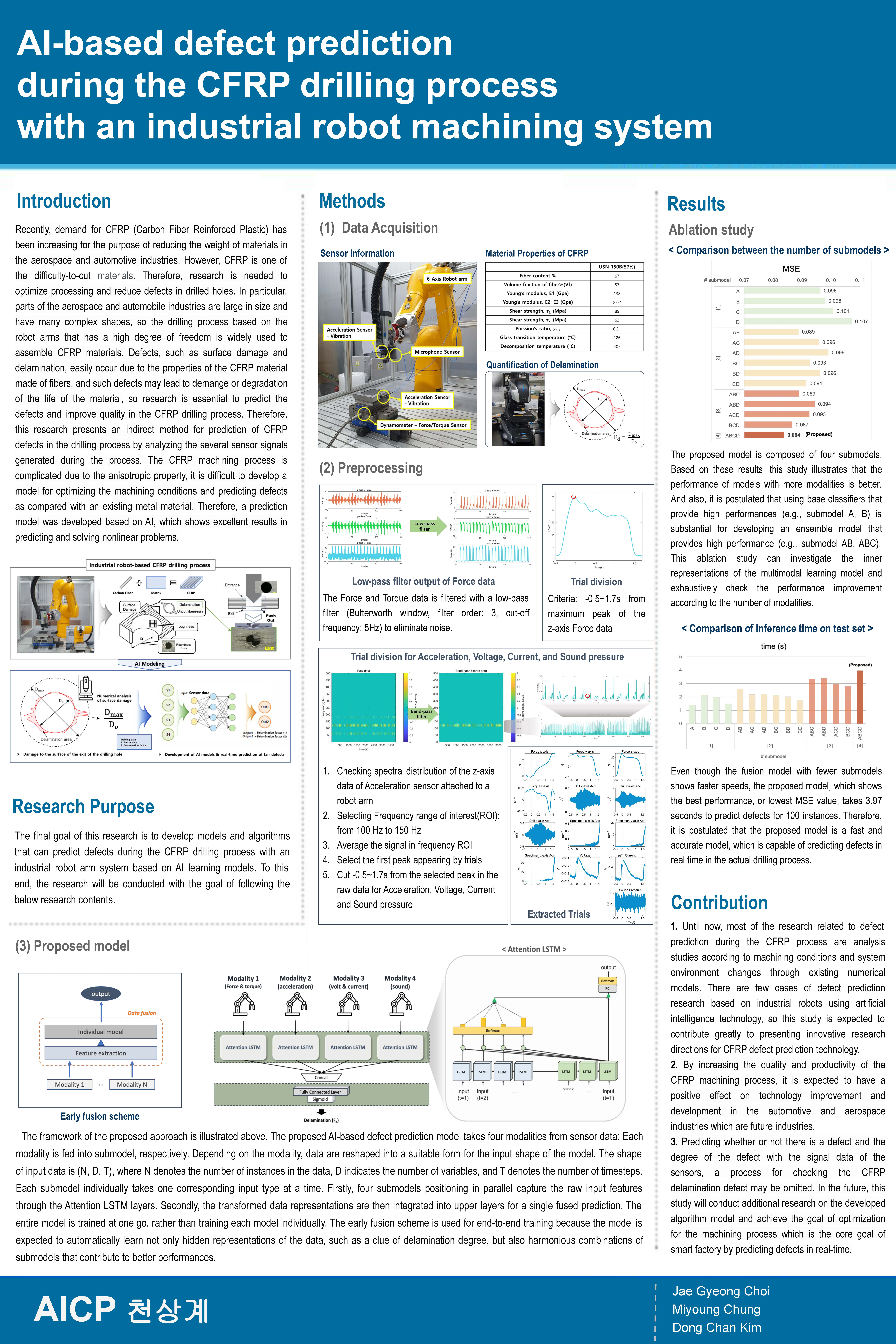
"Won the 2nd prize" (the best prize for Short-term team). AICP challenge is an AI project held in UNIST innovative education center. I am a member of team 'Cheonsang-gye' as a data analyst. We are conducting research with robot sensor data, as the smart factory work environment is becoming prevalent in the aerospace and automobile industry. As the prediction power of the robot arm drilling process is becoming important in that condition, a novel method to predict the delamination on Carbon-fiber Reinforced plastic(CFRP) is developing, and one of the methods is to predict it with an AI algorithm which is unknown yet. We conducted data analysis and applyed a 'multi-modal Neural Network' to the data to predict the delamination more accurately, condidersing all sensor specifications.